히스토그램 평활화
히스토그램 평활화란 히스토그램의 조작을 통한 영상 품질 개선 방식 중의 하나로 가장 대표적인 연산이다.
히스토그램의 분포를 평평하게 만들어 명암의 범위가 늘어나 영상이 이전보다 선명해 진다.
식은 다음과 같다.
\[c(l_{in}) = \sum_{l=0}^{l_{in}} \hat{h}(l) \\
l_{out} = T(l_{in}) = round(c(l_{in}) \times (L-1))\]
여기서 \(c(.)\)는 누적 히스토그램을 나타내고 \(round(.)\)는 반올림 연산을 나타낸다. \(\hat{h}\)는 정규화 히스토그램을 나타낸다.
어떤 명암 \(l\)을 기준으로 생각했을 때, \(l\)보다 작은 명암을 갖는 화소의 비율은 \(1/L\)이어야 한다.
즉, 누적 히스토그램은 비율이 \(1/L\)인 점을 \(l\)로 매핑해준다.
코드구현
코드링크
먼저 사진을 확인해보자. 이번 구현에서는 다음과 같이 어두운 사진을 히스토그램 평활화로 조금 더 밝게 만들것이다.
import cv2
import numpy as np
import matplotlib.pyplot as plt
%matplotlib inline
img = cv2.imread('./data/city.jpg')
plt.imshow(cv2.cvtColor(img,cv2.COLOR_BGR2RGB))
plt.show()
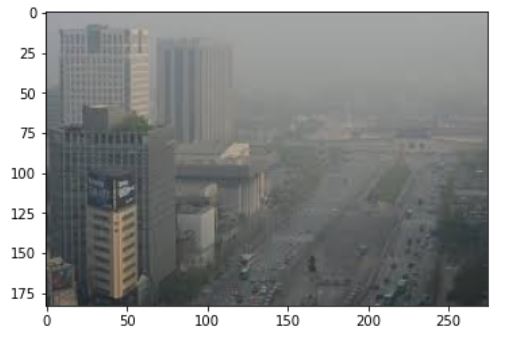
가장 먼저 히스토그램을 구해보자. 아래의 plot을 한 결과는 정규화된 히스토그램이다. 예제에서는 이 값을 저장을 하진 않았다. 혼동하지 말자.
b_hist = np.zeros((256,1))
g_hist = np.zeros((256,1))
r_hist = np.zeros((256,1))
img_flat = np.reshape(img.copy(),(-1,3))
leng=len(img_flat)
for i in range(leng):
b_hist[img_flat[i,0]]+=1
g_hist[img_flat[i,1]]+=1
r_hist[img_flat[i,2]]+=1
fig = plt.figure()
plt.subplot(231)
plt.bar(np.arange(256),b_hist.squeeze()/leng)
plt.xlim([0,256])
plt.subplot(232)
plt.bar(np.arange(256),g_hist.squeeze()/leng)
plt.xlim([0,256])
plt.subplot(233)
plt.bar(np.arange(256),r_hist.squeeze()/leng)
plt.xlim([0,256])
fig.tight_layout()
plt.show()
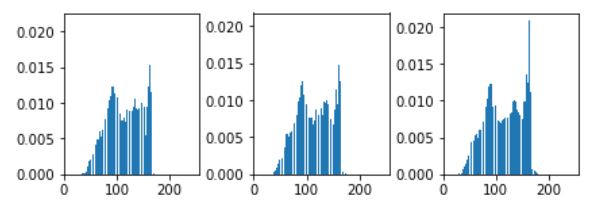
누적 히스토그램은 다음과 같이 순서대로 이전의 값을 더하면 된다. 그리고 이 값을 정규화해 \(L-1\)을 곱해준 후 반올림 한다.
for i in range(1,256):
b_hist[i]+=b_hist[i-1]
g_hist[i]+=g_hist[i-1]
r_hist[i]+=r_hist[i-1]
# 정규화한 값은 저장이 안되어 있으므로 다시 leng으로 나눠 정규화
b_hist= np.uint8(b_hist*255/leng+0.5)
g_hist= np.uint8(g_hist*255/leng+0.5)
r_hist= np.uint8(r_hist*255/leng+0.5)
이제 이미지의 값을 평활화된 히스토그램으로 매핑을 해주면 완성이다.
new = np.zeros_like(img)
for y in range(len(new)):
for x in range(len(new[0])):
new[y,x,0] = b_hist[img[y,x,0]]
new[y,x,1] = g_hist[img[y,x,1]]
new[y,x,2] = r_hist[img[y,x,2]]
plt.subplot(121)
plt.imshow(cv2.cvtColor(img,cv2.COLOR_BGR2RGB))
plt.subplot(122)
plt.imshow(cv2.cvtColor(new,cv2.COLOR_BGR2RGB))
plt.show()
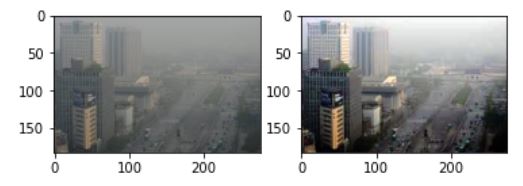
평활화된 이미지의 histogram을 보면 다음과 같다.
b_hist_n = np.zeros((256,1),np.uint8)
g_hist_n = np.zeros((256,1),np.uint8)
r_hist_n = np.zeros((256,1),np.uint8)
img_flat_n = np.reshape(new.copy(),(-1,3))
for i in range(leng):
b_hist_n[img_flat_n[i,0]]+=1
g_hist_n[img_flat_n[i,1]]+=1
r_hist_n[img_flat_n[i,2]]+=1
fig=plt.figure()
plt.subplot(131)
plt.bar(np.arange(256),b_hist_n.squeeze()/leng)
plt.xlim([0,256])
plt.subplot(132)
plt.bar(np.arange(256),g_hist_n.squeeze()/leng)
plt.xlim([0,256])
plt.subplot(133)
plt.bar(np.arange(256),r_hist_n.squeeze()/leng)
plt.xlim([0,256])
fig.tight_layout()
plt.show()
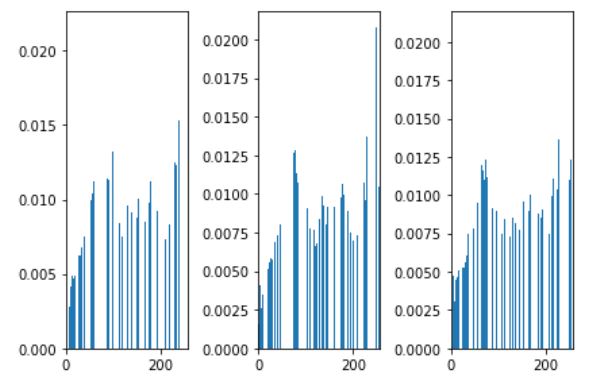
확실히 이전보다 분포가 더 고르게 되어있는 것을 확인할 수 있다.
이 긴과정이 OpenCV의 cv2.equalizeHist 함수 하나면 바로 끝낼 수 있다.
OpenCV를 이용한 예제는 다음과 같이 하면 된다.
cv_new_b = np.expand_dims(cv2.equalizeHist(img[...,0]),-1)
cv_new_g = np.expand_dims(cv2.equalizeHist(img[...,1]),-1)
cv_new_r = np.expand_dims(cv2.equalizeHist(img[...,2]),-1)
cv_new = np.concatenate([cv_new_b,cv_new_g,cv_new_r],-1)
plt.imshow(cv2.cvtColor(cv_new,cv2.COLOR_BGR2RGB))
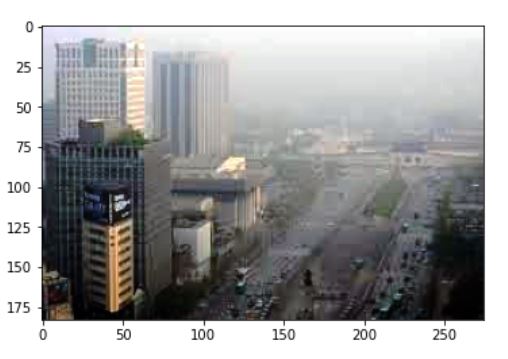
목록으로가기
히스토그램 역투영
히스토그램 역투영은 물체의 모양은 무시하고 단순히 컬러 분포만으로 검출하는 방법이다.
이를 이용해 사람의 얼굴을 검출할 수 있다.
이때 히스토그램은 명안 채널만 이용하는 1차원이 아니라, 최소 2차원 이상을 사용해야 한다. 명안은 조명에 따라 쉽게 변할 뿐만 아니라 얼굴과 비슷한 명암을 갖는 다른 영역이 여러 군데 존재할 가능성이 높아, 명안만 사용하면 피부에 해당하는 영역을 구별하기 어렵다. 따라서 RGB 색상 공간을 HSI 공간으로 변환한 다음, H(색상, Hue)와 S(채도, Saturation) 채널을 사용한다.
histogram의 역투영은 다음과 같이 계산된다.
\[h_{r}(i,j)=min(\frac{\hat{h}_{m}(i,j)}{\hat{h}_{i}(i,j)},0,1) \ , 0 \le j \ , \ i \le q-1\]
여기서 \(\hat{h}_{m}\)은 얼굴 색상을 추출한 정규화된 히스토그램이고 \(\hat{h}_{i}\)은 타겟의 정규화된 히스토그램이다. L의 크기가 클수록 (픽셀의 최대 값) 매우 희소한 공간에 존재하기 때문에 q단계로 줄여서 한다.
식을 이해해 보자면 \(\hat{h}_{m}\)이 \(\hat{h}_{i}\) 이상인 영역을 나타내는 것이다.
코드구현
코드링크
이번 예제는 다음 두 사진을 이용해서 한다. 왼쪽 그림에서 얼굴에 해당하는 히스토그램을 추출하고 오른쪽그림에 역투영을 적용한다.
import numpy as np
import cv2
import matplotlib as mpl
from mpl_toolkits.mplot3d import Axes3D
import matplotlib.pyplot as plt
img = cv2.imread('./data/kim.jpg')
img2 = cv2.imread('./data/girlsgeneration.JPG')
plt.subplot(121)
plt.imshow(cv2.cvtColor(img,cv2.COLOR_BGR2RGB))
plt.subplot(122)
plt.imshow(cv2.cvtColor(img2,cv2.COLOR_BGR2RGB))
plt.show()
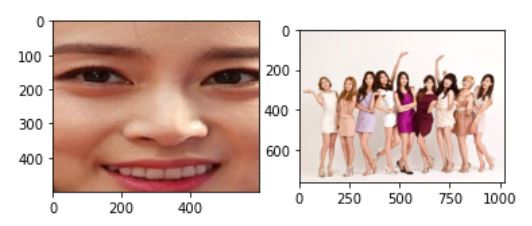
우선 먼저 RGB에서 HSI 색상 영역으로 변환하는 함수를 작성한다. (HSI2RGB는 이곳)
def rgb_to_hsi(img):
heigh,width,channel = img.shape
img_flat = np.reshape(img.copy(),[-1,channel])/255
i = np.mean(img_flat, -1, keepdims=True)
if channel==1:
h = np.zeros_like(img_flat[...,2])
s = np.zeros_like(img_flat[...,2])
else:
r = img_flat[...,2]
g = img_flat[...,1]
b = img_flat[...,0]
mins = np.min(img_flat,axis=1,keepdims=True)
s = 1-mins/i
theta = np.arccos(((r-g)+(r-b))/(np.sqrt((r-g)**2+(r-b)*(g-b))*2))* 180 / np.pi
h = np.expand_dims(np.where(b>g,360-theta,theta),-1)
h/=360
hsi = np.concatenate([h,s,i],-1)
return np.uint8(np.clip(np.reshape(hsi,[heigh,width,channel])*255,0,255))
hsi = rgb_to_hsi(img)
0~255의 값을 가지므로 L은 현재 256, 이 공간을 줄이기 위해 q는 64 정도로 설정하고 히스토그램을 구하자. H 채널과 S 채널, 그리고 3차원 공간에서 히스토그램을 보면 다음과 같다.
L=256
q=64
hs_hist = np.zeros((q,q),np.float)
h_hist = np.zeros((q,1),np.int)
s_hist = np.zeros((q,1),np.int)
src_height, src_width ,_ = img.shape
hsi_flat = np.reshape(hsi.copy(),[-1,3])
for i in range(len(hsi_flat)):
hs_hist[int(hsi_flat[i,0]*q/L)][int(hsi_flat[i,1]*q/L)]+=1
h_hist[int(hsi_flat[i,0]*q/L)]+=1
s_hist[int(hsi_flat[i,1]*q/L)]+=1
hs_hist=hs_hist/(src_width*src_height)
x = np.arange(q)
y = np.arange(q)
xx,yy = np.meshgrid(x,y)
plt.subplot(121)
plt.bar(x,h_hist.squeeze())
plt.xlim([0,q])
plt.subplot(122)
plt.bar(x,s_hist.squeeze())
plt.xlim([0,q])
fig = plt.figure()
ax = fig.gca(projection='3d')
ax.plot_surface(xx,yy,hs_hist.squeeze())
plt.show()
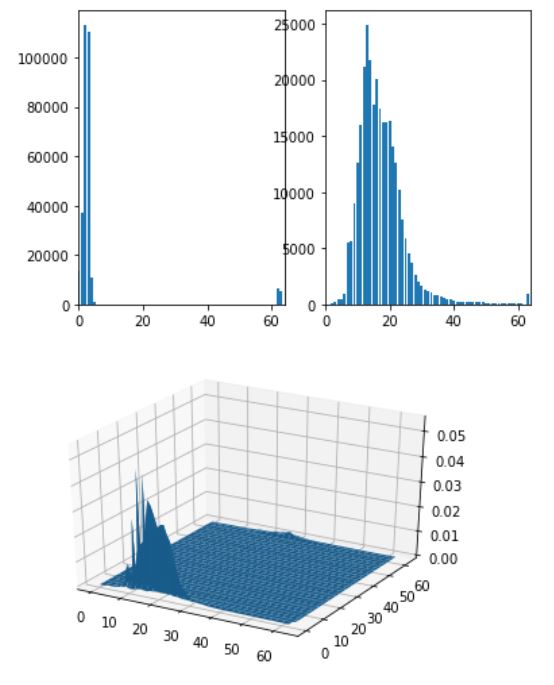
이제 타깃에서도 마찬가지로 히스토그램을 구한다.
dst_height,dst_width,_ = img2.shape
dst_hs_hist = np.zeros((q,q),np.float)
dst_hsi = rgb_to_hsi(img2)
hsi_flat = np.reshape(dst_hsi.copy(),[-1,3])
for i in range(len(hsi_flat)):
dst_hs_hist[int(hsi_flat[i,0]*q/L)][int(hsi_flat[i,1]*q/L)]+=1
dst_hs_hist=dst_hs_hist/(dst_width*dst_height)
마지막으로 타겟의 HSI 영역에서 히스토그램을 역투영하면 얼굴과 유사한 영역이 검출된다.
dst = np.zeros([dst_height,dst_width])
for y in range(dst_height):
for x in range(dst_width):
h=int(dst_hsi[y, x,0] * q / L)
s=int(dst_hsi[y,x,1]*q/L)
dst[y][x]=np.clip(hs_hist[h,s]/dst_hs_hist[h,s],0,1)*255
plt.subplot(121)
plt.imshow(cv2.cvtColor(img2,cv2.COLOR_BGR2RGB))
plt.subplot(122)
plt.imshow(dst, cmap='gray')
plt.show()
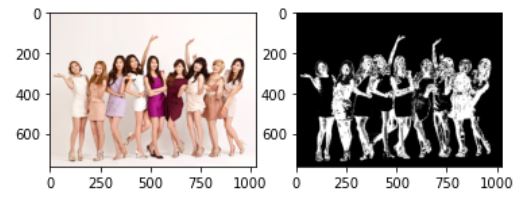
OpenCV에서는 q를 사용하지는 않고 간단하게 구할 수 있다.
OpenCV에서는 RGB2HSI가 지원되지 않는다. 따라서 HSV를 이용해 구현을 한다. (HSI와 HSV의 수식이 조금 달라서 값이 조금 차이가 난다.)
cv_hsv = cv2.cvtColor(img,cv2.COLOR_BGR2HSV)
cv_hsv2 = cv2.cvtColor(img2,cv2.COLOR_BGR2HSV)
cv_hs_hist = cv2.calcHist([cv_hsv], [0,1], None, [180,256], [0,180,0, 256])
cv2.normalize(cv_hs_hist,cv_hs_hist,0,255,cv2.NORM_MINMAX)
cv_dst=cv2.calcBackProject([cv_hsv2],[0,1], cv_hs_hist,[0,180,0,256],1) # 역투영함수
disc = cv2.getStructuringElement(cv2.MORPH_ELLIPSE,(5,5)) # smooding mask
cv2.filter2D(cv_dst,-1,disc,cv_dst) # smoothing
plt.subplot(121)
plt.imshow(cv2.cvtColor(img2,cv2.COLOR_BGR2RGB))
plt.subplot(122)
plt.imshow(cv_dst, cmap='gray')
plt.show()
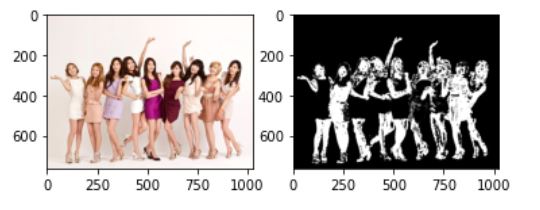
추가적으로 검출된 영역을 다음과 같이 하면 검출 영역의 색상을 띄우는 것이 가능하다.
_,thr = cv2.threshold(cv_dst,5,255,0)
thr = cv2.merge((thr,thr,thr))
cv_dst = cv2.bitwise_and(img2,thr)
plt.subplot(121)
plt.imshow(cv2.cvtColor(img2,cv2.COLOR_BGR2RGB))
plt.subplot(122)
plt.imshow(cv2.cvtColor(cv_dst,cv2.COLOR_BGR2RGB))
plt.show()
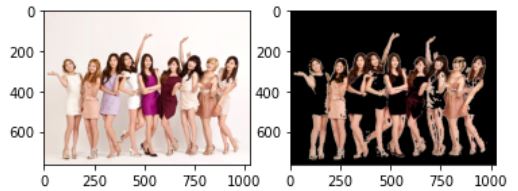
히스토그램
히스토그램이란 영상의 명암값이 나타난 빈도수로, [0,L-1] 사이의 명암값 각각이 영상에 몇 번 나타나는지를 표시한다.
Opencv를 이용해 이미지를 불러오는 경우 0~255로 명암값을 나타낸다. 즉 [0,255] 사이의 명암값 각각이 영상에 몇 번 나타나는지를 표시한다.
히스토그램의 여러 용도 중 하나는 영상의 특성을 파악하는 것이다. 히스토그램이 왼쪽으로 치우처져 있으면 어두운 영상, 오른쪽으로 치우처져 있으면 밝은 영상이다.
코드 구현
코드링크
코드의 구현은 간단하다. 각 픽셀의 노출 횟수를 카운트하면 된다.
예제는 유명한 레나사진을 사용할 것이다. OpenCV의 함수를 이용하면 bgr 순서로 채널이 되어 있기때문에 cv2의 imshow를 이용하는 게 아닐때는 rgb로 순서를 바꾸는게 좋다.
import cv2
import numpy as np
import matplotlib.pyplot as plt
%matplotlib inline
img = cv2.imread('./data/lena.jpg')
plt.imshow(cv2.cvtColor(img,cv2.COLOR_BGR2RGB))
plt.show()
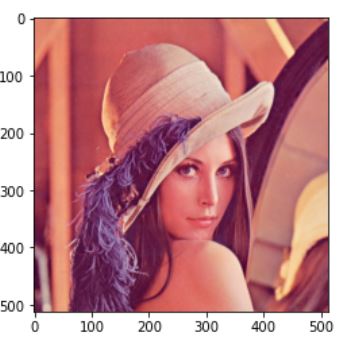
numpy를 이용해 0으로 초기화된 리스트를 선언하고 각 채널의 명암값을 카운트한다.
r_hist = np.zeros((256))
b_hist = np.zeros((256))
g_hist = np.zeros((256))
im_flat = np.reshape(img.copy(),(-1,3))
leng = len(im_flat)
for i in range(leng):
b_hist[im_flat[i][0]]+=1
g_hist[im_flat[i][1]]+=1
r_hist[im_flat[i][2]]+=1
matplotlib을 이용하면 count된 횟수를 볼 수 있다.
fig = plt.figure()
plt.subplot(131)
plt.plot(b_hist,color='b')
plt.xlim([0,256])
plt.subplot(132)
plt.plot(g_hist,color='g')
plt.xlim([0,256])
plt.subplot(133)
plt.plot(r_hist,color='r')
plt.xlim([0,256])
fig.tight_layout() # 그래프가 겹치는 것을 방지 할 수 있음
plt.show()
OpenCV에서는 cv2.calcHist로 단숨에 계산할 수 있다.
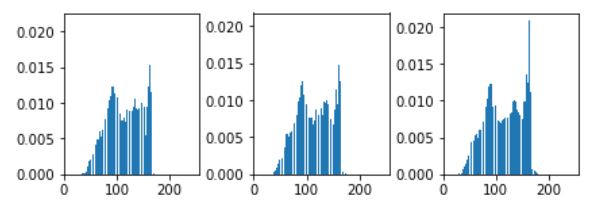
누적 히스토그램은 다음과 같이 순서대로 이전의 값을 더하면 된다. 그리고 이 값을 정규화해 \(L-1\)을 곱해준 후 반올림 한다.
## using cv
fig = plt.figure()
color = ['b','g','r']
for i in range(3):
hist = cv2.calcHist([img], [i], None, [256], [0, 256])
plt.subplot(131+i)
plt.plot(hist,color=color[i])
plt.xlim([0,256])
fig.tight_layout()
plt.show()
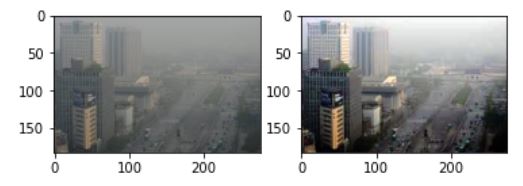
16 May 2020
•
Paper
1. INTRODUCTION
More …
15 May 2020
•
TIL
오늘은 다행히 무거운 코드가 없어서 주피터로 감당 가능했다.
앞으로 주말에는 논문 구현하는 연습을 해야겠다. 우선 논문을 어떻게 읽는가에 대한 논문이 있는데 그거 먼저 올려야지
TID
- 핸즈온 머신러닝 4~4.3장
- 종만북-algospot 1문제, 예제 2개
TODO list